Segmentation Based Multi-Cue Integration for Object Detection
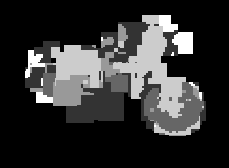
This paper proposes a novel method for integrating multiple local cues, i.e. lo- cal region detectors as well as descriptors, in the context of object detection. Rather than to fuse the outputs of several distinct classifiers in a fixed setup, our approach implements a highly flexible combination scheme, where the con- tributions of all individual cues are flexibly recombined depending on their ex- planatory power for each new test image. The key idea behind our approach is to integrate the cues over an estimated top-down segmentation, which allows to quantify how much each of them contributed to the object hypothesis. By combining those contributions on a per-pixel level, our approach ensures that each cue only contributes to object regions for which it is confident and that potential correlations between cues are effectively factored out. Experimental results on several benchmark data sets show that the proposed multi-cue combi- nation scheme significantly increases detection performance compared to any of its constituent cues alone. Moreover, it provides an interesting evaluation tool to analyze the complementarity of local feature detectors and descriptors.