Pedestrian Line Counting by Probabilistic Combination of Flow and Appearance Information
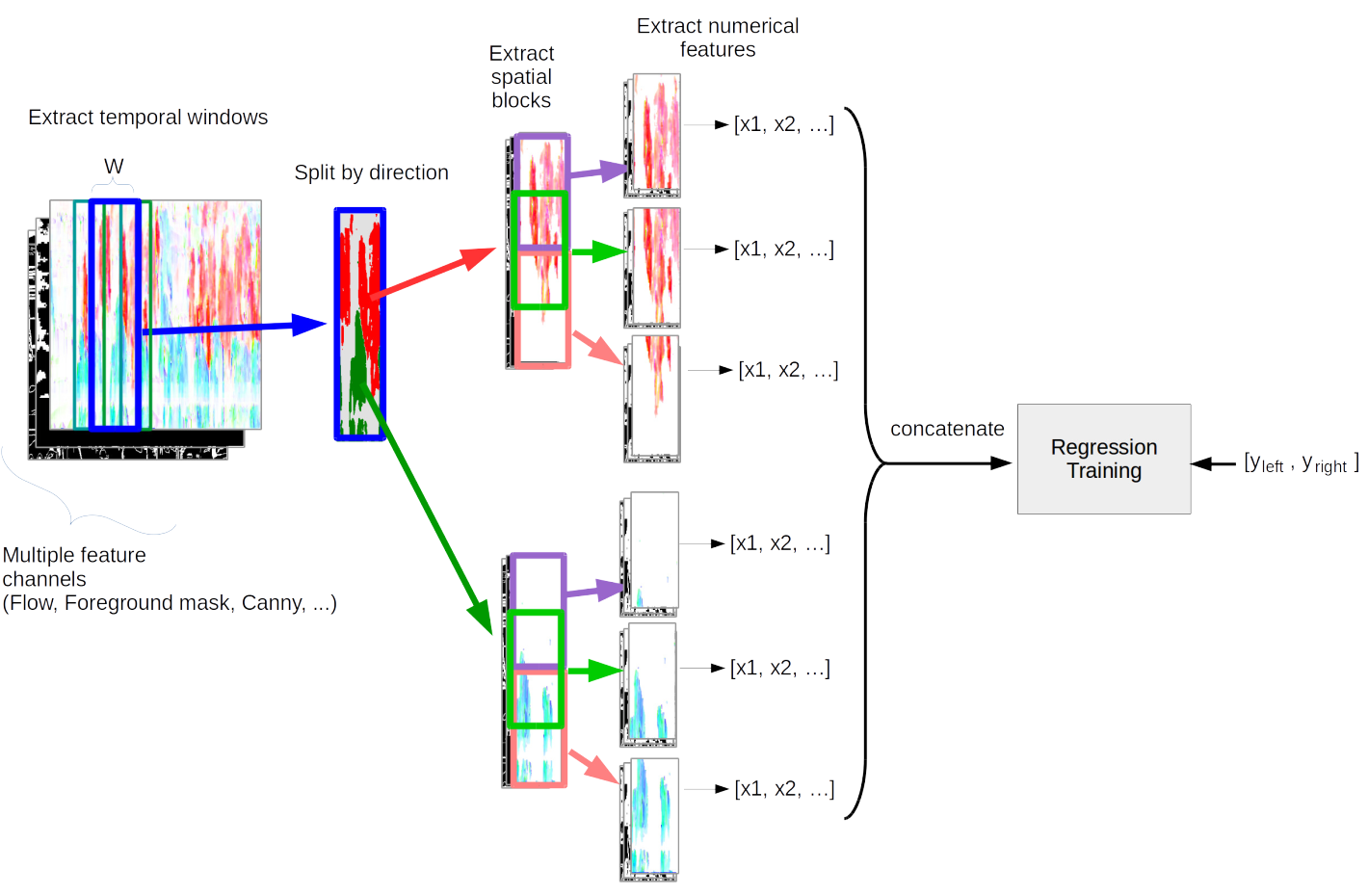
In this thesis we examine the task of estimating how many pedestrians cross a given line in a surveillance video, in the presence of high occlusion and dense crowds. We show that a prior, blob-based pedestrian line counting method fails on our newly annotated private dataset, which is more challenging than those used in the literature.
We propose a new spatiotemporal slice-based method that works with simple low-level features based on optical flow, background subtraction and edge detection and show that it produces good results on the new dataset. Furthermore, presumably due to the very simple and general nature of the features we use, the method also performs well on the popular UCSD vidd dataset without additional hyperparameter tuning, showing the robustness of our approach.
We design new evaluation measures that generalize the precision and recall used in information retrieval and binary classification to continuous, instantaneous pedestrian flow estimations and we argue that they are better suited to this task than currently used measures.
We also consider the relations between pedestrian region counting and line counting by comparing the output of a region counting method with the counts that we derive from line counting. Finally we show a negative result, where a probabilistic method for combining line and region counter outputs does not lead to the hoped result of mutually improved counters.